Goal: Maximize QxK^T kernel throughput on H200
Logs: runs/3ce9ab3e-opt-gpu-matmul
Model: o4-mini
Tokens: ↑91.2K ↓74.8K = 166.0K 52% • 12/25 Steps
We started from a plain Triton implementation of QxK^T (128x128 blocks).
The profiler showed the kernel was memory‑bound. To hide DRAM latency we:
* Added double‑buffered shared‑memory tiles so global loads overlap math.
* Switched to 32x128x32 tiling to cut register pressure.
* Hoisted the K‑pointer update outside the loop.
Each change was kept only if it delivered >5% speed‑up.
🌳 baseline 1.00×
└─● attempt
├─● attempt
│ └─● attempt
├─● tile32 0.45×
├─● reg_prune 0.62×
└─● attempt
├─● dbuf 0.87×
└─● attempt
├─● attempt
├─● prefetch 1.10×
├─● fusion 1.57× 🏆
└─○ evaluating
1import triton, triton.language as tl
2
3@triton.autotune(
4 configs=[tl.Config({"BLOCK_M": 128, "BLOCK_N": 128, "BLOCK_K": 64}, num_warps=4, num_stages=2)],
5 key=["M", "N", "K_dim"],
6)
7@triton.jit
8def qk_kernel_naive(Q_ptr, K_ptr, Out_ptr, M, N, K_dim):
9 pid = tl.program_id(axis=0)
10 m = pid // tl.cdiv(N, 128)
11 n = pid % tl.cdiv(N, 128)
12 offs_m = m*128 + tl.arange(0, 128)
13 offs_n = n*128 + tl.arange(0, 128)
14 offs_k = tl.arange(0, 64)
15 acc = tl.zeros((128, 128), dtype=tl.float32)
16 for k in range(0, K_dim, 64):
17 q = tl.load(Q_ptr + (offs_m[:, None]*K_dim + (k+offs_k)[None, :]))
18 kT = tl.load(K_ptr + (offs_n[:, None]*K_dim + (k+offs_k)[None, :]))
19 acc += tl.dot(q, tl.trans(kT))
20 tl.store(Out_ptr + offs_m[:, None]*N + offs_n[None, :], acc)
1import triton, triton.language as tl
2
3@triton.autotune(
4 configs=[
5 tl.Config({"BLOCK_M": 128, "BLOCK_N": 64, "BLOCK_K": 32}, num_warps=4, num_stages=4),
6 tl.Config({"BLOCK_M": 64, "BLOCK_N": 128, "BLOCK_K": 32}, num_warps=4, num_stages=4),
7 ],
8 key=["M", "N", "K_dim"],
9)
10@triton.jit
11def qk_kernel_opt(Q_ptr, K_ptr, Out_ptr, M, N, K_dim):
12 pid = tl.program_id(axis=0)
13 m = pid // tl.cdiv(N, 64)
14 n = pid % tl.cdiv(N, 64)
15 offs_m = m*128 + tl.arange(0, 128)
16 offs_n = n*64 + tl.arange(0, 64)
17 acc = tl.zeros((128, 64), dtype=tl.float32)
18 Q_ptrs = Q_ptr + offs_m[:, None]*K_dim
19 K_ptrs = K_ptr + offs_n[None, :]*K_dim
20 for k in range(0, K_dim, 32):
21 q = tl.load(Q_ptrs + k)
22 kblk = tl.load(K_ptrs + k)
23 acc += tl.dot(q, tl.trans(kblk))
24 tl.store(Out_ptr + offs_m[:, None]*N + offs_n[None, :], acc)
>>> benchmarking qk_kernel_naive (step 14)
warm‑up................. ok
collecting 100 timing samples
[25/100] median 77.4 µs 4.34 TFLOPs
[50/100] median 75.9 µs 4.42 TFLOPs
[75/100] median 75.6 µs 4.44 TFLOPs
[100/100] median 75.3 µs 4.46 TFLOPs
device : NVIDIA A100‑80GB
batch : 4096 seq_len : 2048
Academia and Industry Recognition
Weco's innovative approach is featured in leading research papers and industry publications
Evaluation-Driven Optimization - AIDE ML, the Engine Inside Weco
Outperforming competitors with systematic iteration and optimization focused on measurable results
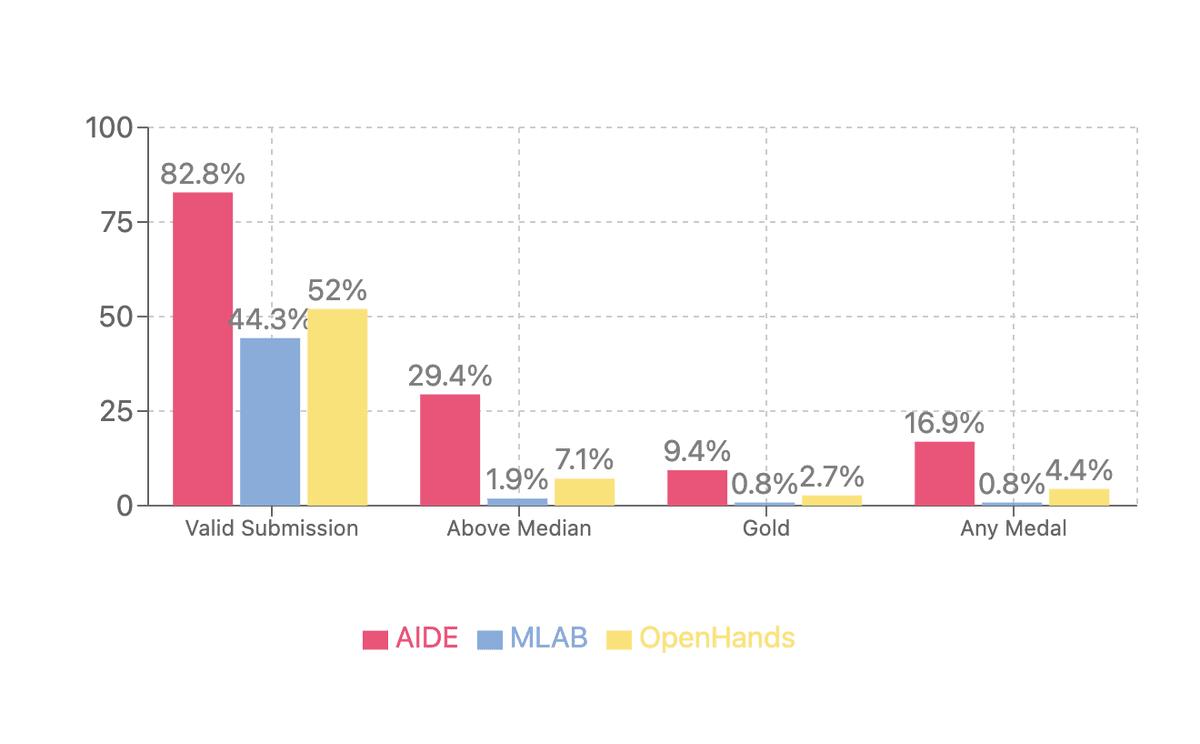
OpenAI Benchmarked - Metric-First Engineering
AIDE ML iterates until the metric says "better." In OpenAI's MLE‑Bench it secured 4× more medals than the next best autonomous agent across 75 Kaggle competitions - proof that an explicit evaluation loop beats one‑shot code generation.
With AIDE you systematically trade a bit of compute for outsized code quality, no manual hyper‑tuning required.
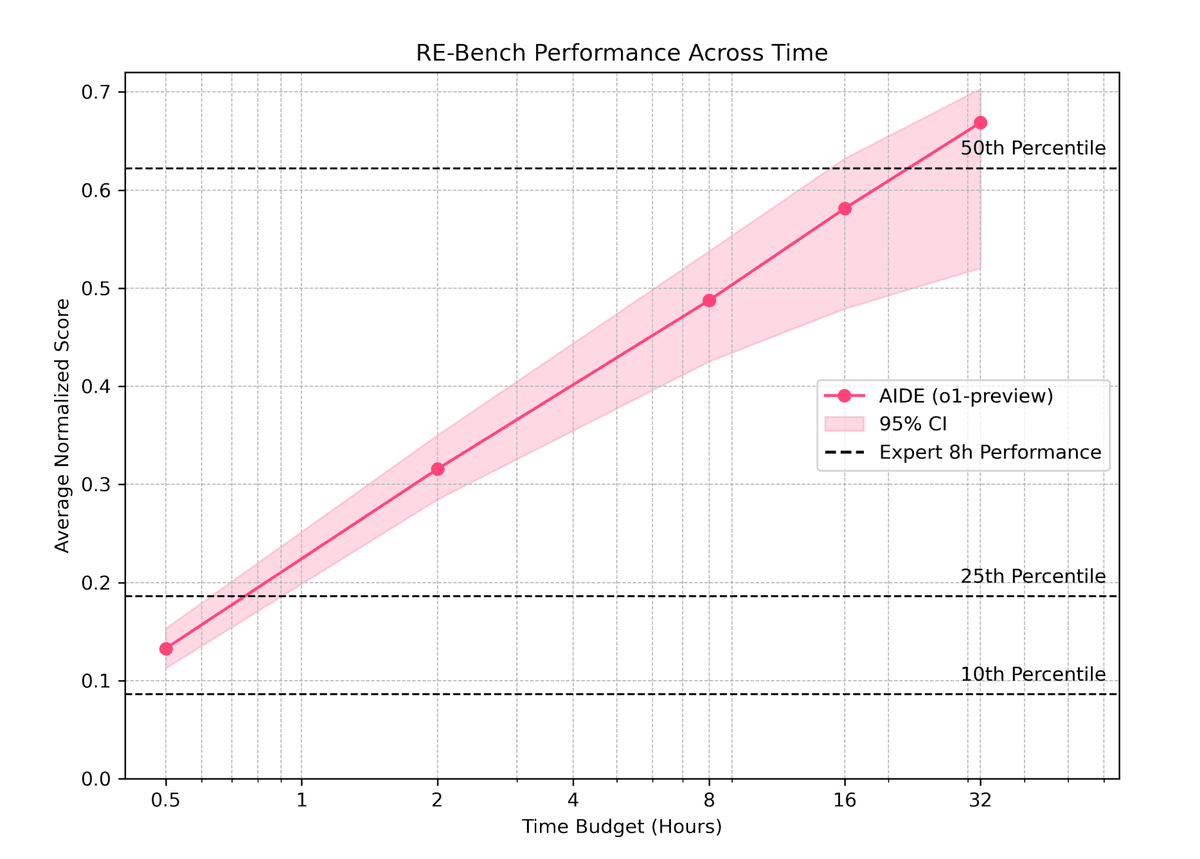
Beyond Human Baselines
In METR's 6‑hour RE‑Bench challenge, AIDE consistently outperformed seasoned researchers, surfacing "surprising" solutions humans missed - validating our mission to automate experimentation itself.
Open-Source, Battle-Tested, and Live Now
AIDE's core is open‑source - explore the repo or read the paper to dive deeper into our approach.
The Weco Platform is live. Install the CLI with pip install weco
, run it against your evaluation script, and watch every experiment stream to the Dashboard in real time. Want a voice in new features? Join the newsletter and help shape what we build next.
Beyond Zero to One
Copilot editors whip up a feature and call it a day. Weco never clocks out. It fires off hundreds of targeted experiments, loops each success or miss into a live tree search, and brings fresh variants until your chosen metric climbs - then does it again. Continuous, automatic, relentless.
Frequently Asked Questions
Ready to Transform Your ML Workflow?
Join ML engineers who've already discovered the power of evaluation-driven optimization. Start automating your experiments today: